Abstract
Introduction: The goal of this study was to elucidate a link of brain-derived factor (BDNF) Val66Met gene with combined 6-month clinical end points in post-myocardial infarction patients.
Methods: 256 post-myocardial infarction patients who underwent primary coronary intervention were enrolled in the study. Variants of Val66Met gene BDNF were identified by real-time chain reaction at baseline.
Results: The combined clinical end points (major cardiovascular events and hospitalization) were determined in 61 (23.8%) post-STEMI patients; consequently, 195 (76.2%) patients did not meet the events. linear regression revealed that predictors for combined clinical end points were peak TnI levels, NT-proBNP, SYNTAX score, TIMI score, obesity, left ventricular ejection fraction, and 66ValMet+66MetMet in BDNF gene. The cumulative clinical outcomes (major adverse cardiac events and admission) were determined in 61 (23.8%) patients. Kaplan-Meier curves demonstrated that 66ValVal of BDNF gene was significantly associated with the low number of combined end points.
Conclusion: The Val66Met in BDNF gene independently predicted 6-month combined clinical end points in post-myocardial infarction patients.
Introduction
Recurrent major adverse cardiac events (MACEs) and heart failure (HF) remain the most common causes of premature cardiovascular (CV) mortality amid post-ST-segment elevation myocardial infarction (STEMI) patients, regardless of wide implementation of early re-vascularization strategies including primary percutaneous coronary intervention (PPCI)1, 2. In fact, conventional pure approaches based on clinical assessment, transthoracic echocardiography and biomarkers of myocardial necrosis are insufficient today to catch vulnerable post-STEMI patients after successful PPCI3. Although there are several biomarkers (natriuretic peptides, high sensitive cardiac troponins, soluble ST2, etc.) that are designed for risk stratification among patients with STEMI4, 5, 6, the risk assessment and prediction of poor clinical outcomes after successful competed PPCI in post-STEMI patients are uncertain7. There are several limitations that could decrease the predictive value of conventional biomarkers in post-STEMI individuals, such as older age, morbid obesity, diabetes mellitus (DM), preserved or normal left ventricular (LV) ejection fraction (EF), co-existing kidney insufficiency and atrial fibrillation8, 9, 10.
Neurotrophins are a superfamily of regulatory proteins which regulate proliferation, differentiation, survival and plasticity of neurons11. Brain-derived neurotropic factor (BDNF) was previously found as one of the neurotrophins with proliferative, cholinergic, serotoninergic and dopaminergic activities, and is predominantly synthesized in central and peripheral neurons12. Later, an expression of mRNA BDNF was found in myocardium, vessel vasculature, skeletal muscles, parenchymal organs (including lung, spleen and kidney), visceral epithelial cells, and mature and progenitor endothelial cells13, 14, 15. Previous animal and clinical studies have revealed that BDNF through an activation of nuclear factor kB receptors mediates endothelial cell survival and neoangiogenesis, reduces p75-mediated apoptosis of cardiac myocytes, enhances endothelial function, regulates blood flow in ischemic myocardium, and improves LV function after ischemic injury, thereby providing cardioprotective effects16, 17.
Observational and clinical investigations have shown strong inverted associations between BNDF levels in peripheral blood and cognitive dysfunction in HF patients, and CV risk in individuals with known DM, hypertension, cardiomyopathy, stable coronary artery disease and STEMI18, 19, 20, 21. However, circulating levels of BDNF exhibited a close correlation with single nucleotide polymorphism (SNP) in BDNF gene that was associated with a replacement of valine to methionine in the 66 position of codon (Val66Met). It has been suggested that this SNP Val66Met affects the intracellular processing of the peptide and leads to a decline in the secretion of BDNF, resulting in lowered susceptibility to ischemia and myocardial injury22, 23. The aim of the study was to elucidate a link of BDNF Val66Met gene with cumulative 6-month clinical end points in post-myocardial infarction patients.
Material and Methods
Patient population
From 2016 August to 2019 February, 320 patients underwent selective coronary angiography due to suspected acute STEMI. Acute STEMI was diagnosed in accordance to the European Society of Cardiology (ECS) recommendation (2017)24. The study flow chart is shown in Figure 1. We identified 256 acute STEMI individuals who underwent PPCI with an implantation of bare-metal stent “COMMANDER” manufactured by “Alvimedica” (Turkey) in culprit artery (1 to 4 stents onto ischemic-related arteries) and discharged with post-PPCI TIMI III score from the hospital. All patients were treated with current adjuvant care24.
Coronary angiography
We used radial or femoral vascular access and two-to-three orthogonal projections to receive angiograms at baseline. A digital X-ray system "Integris Allura-9" (Philips Healthcare, Eindhoven, Netherlands) was used to visualize coronary anatomyto visualize coronary anatomy. Ultravist-370 (Baier Pharma, GmbH, Germany) was the contrast giving automatically through the injector.
Ethical declaration
All patients enrolled in the study gave voluntary informed consent to participate. The study was approved by the local ethics committee (Protocol №8, 29.08.2016). The study had been carried out in accordance with the 1964 Helsinki declaration.
Sample size calculation
We calculated sample size by taking into consideration the effect size estimation (0.99), type II error (0.2), type I error (0.05), expected mortality rate of 7%, and one-year mortality rate of 14%25. The sample size was 250 individuals.
Determination of risk factors and co-morbidities
DM was diagnosed as serum level of fasting glucose of > 7.0 mmol/L, 2-h postprandial glucose serum level of > 11.1 mmol/L, or current therapy of anti-diabetic drugs26. Hypercholesterolemia (HCE) hypertension was diagnosed in accordance with current recommendation27, 28. HF was diagnosed according to current ECS clinical guidelines29. Information of other diseases and medical history were obtained by investigators through a review of medical history or direct contact with the patient’s general practitioner.
Echocardiography examination
Transthoracic B-mode echocardiography and Tissue Doppler Imaging were carried out using 3.5 MHz phase probe with “Aplio 500” (TUS-A500, Toshiba Inc., Japan) at baseline. LVEF were measured by Simpson's method. LV global longitudinal strain (e`) and early transmitral velocity (E) were measured by tissue Doppler imaging technique and impulse transmitral Doppler regime, respectively.
Determination of STEMI prognosis
We used the TIMI score and the GRACE score to validate prognostic capacity after STEMI30, 31.
SYNTAX score determination
SYNTAX score (SS) was calculated by an experienced interventional cardiologist accordingly32.
Determination of endpoints
The primary endpoint was MACEs in combination with hospitalization that occurred during 6-month period post-discharge period. MACEs included CV death, recurrent angina, and newly diagnosed HF. The endpoint was ascertained by personal or phone contact with a general practitioner or staff of the hospital in which the patient had been admitted.
Calculation of glomerular filtration rate
The Chronic Kidney Disease Epidemiology Collaboration (CKD-EPI) equation was used to calculate glomerular filtration rate (GFR)33.
Collection of blood samples
Venous blood samples were collected from the patients using vacutainers at baseline and at the end of the study. After 30-minute centrifugation, the serum was collected and then stored at -700C until transfer to the laboratory of GI “L.T.Malaya TNI NAMSU”. Hematology and biochemistry, including lipid profiles and fasting glucose, were provided for each patient.
Serum levels of troponin I (TnI) were measured by chemo-luminescent immunoassay method on the biochemical analysator (Humalyser 2000, Mannheim, Germany). The average of the TnI level was 0.5-50 ng/mL.
N-terminal fragment of brain natriuretic peptide (NT-proBNP) was evaluated by use of a commercially available kit manufactured by R&D Systems GmbH (Wiesbaden-Nordenstadt, Germany). The average of the NT-proBNP level was 10-12000 pg/mL.
SNP Val66Met (rs6265) in BDNF gene determination
The extraction of DNA was executed per the conventional protocol for a commercial set (TacMan TMSNP Genotyping Assays; Thermo Fisher Scientific Assay IDC_11592758_1). The BDNF genetic variants were evaluated by real-time polymerase chain reaction (PCR). Primers used in the BDNF Val66Met (rs6265) polymorphism assay were as follows: CCTACAGTTCCACCAGGTGAGAAGAGTG (forward), TCATGGACATGTTTGCAGCATCTAGGTA (reverse).
Statistics
Baseline characteristics are presented as mean ± standard deviation (SD) or median (IQR) for continuous variables and proportions for categorical variables. Chi-square test or Mann-Whitney U test and T-test were used to compare the categorical or normally-distributed data, respectively. Hardy–Weinberg Equilibrium was conducted to estimate allele frequencies. Spearman correlation analysis was performed to investigate the relationship between circulating levels of cardiac biomarkers, co-morbidities, and BDNF gene SNP. Univariate and multiple variate linear regression analyses were done; factors that predict the combined endpoint were determined. The beta coefficient, standard error (SE), odds ratio (OR), and 95% confidence interval (CI) for predictors were evaluated. We also checked whether five common subject-level gene models (recessive, multiplicative, additive, dominant, and over-dominant models) were related to the explanatory variables34. We also used area under curve (AUC), integrated discrimination indices (IDI), and net-reclassification improvement (NRI) for prediction performance analyses. Significant differences between variables were set at p-value < 0.05.
Results
The combined clinical endpoints (MACEs and hospitalization) were determined in 61 (23.8%) post-STEMI patients; consequently, 195 (76.2%) patients did not meet the events. General clinical characteristics of the entire patient population enrolled in the study and both cohorts (with MACEs and free MACEs) are reported in Table 1. The entire population consisted of participants with mean age of 58.76 years old, with male/female proportion of 77.3%/22.7%, with CV risk factors including hypertension (52%), type 2 DM (T2DM) (19.5%), hypercholesterolemia (63.7%), obesity (37.5%), and smoking (34.9%). We did not find significant differences between both cohorts of the post-STEMI patients in terms of demographics, glomerular filtration rate, and CV risk factor presentation, except in the incidence of T2DM and hypertension, which were more frequently seen in patients who met MACEs than in individuals who were free of MACEs (P = 0.032 and P = 0.0008, respectively). Therefore, participants with MACEs were older that those who did not meet MACEs (P = 0.027).
Variables | Entire STEMI population (n = 256) | Patients who met MACEs (n = 61) | Patients who did not meet MACEs (n = 195) | χ2,р |
Age, years, M (SD) | 58.76±9.85 | 61.07±9.39 | 58.13±10.02 | 0.027 |
Male, n (%) | 198(77.3) | 42(68.9) | 156(80.0) | 0.070 |
Female, n (%) | 58(22.7) | 19(31.1) | 39(20.0) | |
Hypertension, n (%) | 133(52.0) | 39(63.9) | 94(48.2) | 0.032 |
T2DM, n (%) | 50(19.5) | 21(34.4) | 29(14.9) | 0.0008 |
Smoking, n (%) | 89(34.9) | 27(44.3) | 62(31.8) | 0.074 |
HCE, n (%) | 163(63.7) | 42(68.9) | 121(62.1) | 0.335 |
BMI > 30 kg/m2, n (%) | 96(37.5) | 24(39.3) | 72(36.9) | 0.733 |
GFR, ml/min/1.73 m2 | 104.67±27.56 | 103.68±27.77 | 107.50±26.96 | 0.389 |
Circulating biomarkers | ||||
Peak TnI, ng/ml | 18.4 [5.44-87.3] | 21.56 [11.55-99.45] | 13.18 [5.97-68.5] | 0.038 |
NT-proBNP, pg/mL | 246.81 [26.78 – 610.97] | 415.12 [74.45-1305,42] | 202.43 [54.48-802.60] | 0.001 |
TC, mmol/l | 4.97[4.00-5.75] | 5.13[4.26-5.87] | 4.89[3.97-5.68] | 0.192 |
HDL, mmol/l | 1.09 [0.90-1.28] | 1.12 [0.94-1.28] | 1.08 [0.90-1.28] | 0.534 |
LDL, mmol/l | 3.00[2.11-3.71] | 3.20[2.45-3.98] | 2.86[2.06-3.63] | 0.078 |
TG, mmol/l | 1.53 [1.17-2.02] | 1.48 [1.17-1,83] | 1.57 [1.17-2.06] | 0.455 |
SNP polymorphism of BDNF gene | ||||
66ValVal, n (%) | 190(74.2) | 42(68.9) | 148(75.9) | 0.272 |
66ValMet+66MetMet, n (%) | 66(25.8) | 19(31.1) | 47(24.1) | |
Concomitant medications | ||||
Beta-blockers, n (%) | 256 (100) | 61 (100) | 195 (100) | 1.00 |
ACEI / ARBs, n (%) | 245 (95.7) | 58 (95.1) | 187 (95.9) | 0.98 |
Clopidogrel /Ticagrelor, n (%) | 252 (98.4) | 58 (95.1) | 194 (99.5) | 0.99 |
Statins, n (%) | 256 (100) | 61 (100) | 195 (100) | 1.00 |
MCRAs, n (%) | 178 (69.5) | 43 (70.5) | 135 (69.2) | 0.94 |
Metformin, n (%) | 32 (12.5) | 10 (16.4) | 22 (11.3) | 0.046 |
Loop diuretics, n (%) | 71 (27.7) | 20 (32.7) | 51 (26.2) | 0.12 |
The patients who met MACEs exhibited higher peak serum levels of troponin I and circulating levels of NT-proBNP than those who had no MACEs. Significant differences in lipid profiles among both patient cohorts were not found.
The observed frequency of Val66Met BDNF genotype in post-STEMI patients was as follows: 66ValVal — 74.2% and 66ValMet+66MetMet — 25.8%. Genotype frequency matched to Hardy–Weinberg equilibrium (χ2 = 0.17, P > 0.05). There were not differences between both cohorts in terms of phenotype of BDNF (P = 0.272).
All post-STEMI patients were treated according to contemporary clinical protocol and there were not significant differences in concomitant medications, except for metformin which was prescribed frequently in the patient cohort with MACEs (P = 0.046).
We found that TIMI risk in patients with MACEs was significantly higher in comparison with individuals who had no combined endpoint (P = 0.046) (Table 2). Nevertheless, the number of participants in both cohorts having 22 – 32 SYNTAX score points and ≤ 22 SYNTAX score points did not differ, but the proportion of the patients with MACEs having > 32 SYNTAX score points occurred frequently when compared with those who did not meet the endpoint. Additionally, total GRACE score points were similar in both patient cohorts (P = 0.294).
Variables | Entire STEMI population (n = 256) | Patients who met MACEs (n = 61) | Patients who did not meet MACEs (n = 195) | χ2,р |
STEMI scores | ||||
TIMI risk score, point | 6 [4-7] | 8 [5-9] | 6 [4-8] | 0.046 |
Total SYNTAX score, point | 28.7±6.15 | 27.54±6.41 | 25.65±8.82 | 0.134 |
> 32 points, n (%) | 76 (42.9) | 41 (54.6) | 35 (34.3) | 0.011 |
22 - 32 points, n (%) | 79 (44.6) | 38 (50.7) | 41 (40.2) | 0.167 |
≤ 22 points, n (%) | 22 (12.4) | 10 (13.3) | 12 (11.8) | 0.765 |
Total GRACE Score, points | 150 (120-172) | 143 (117-170) | 152 (119-176) | 0.294 |
STEMI localization | ||||
Anterior, n (%) | 126(49.2) | 30(49.2) | 96(49.2) | 0.995 |
Posterior, n (%) | 113(44.1) | 22 (36.1) | 91(50.8) | |
Lateral, n (%) | 17 (6.6) | 9 (14.8) | 8 (4.1) | |
Culprit coronary arteries | ||||
One vessel injury, n (%) | 96(37.5) | 15(24.6) | 81(41.5) | 0.017 |
Two vessels injury, n (%) | 69 (26.9) | 19(31.1) | 51(26.2) | 0.445 |
Three and more vessels injury, n (%) | 70 (27.3) | 22(36.1) | 48(24.6) | 0.080 |
Quantity of stenosis | 2.0 [1.0-4.0] | 2.0 [1.0-4.0] | 2.0 [1.0-4.0] | 1.0 |
Left anterior descending injury, n (%) | 185(72.3) | 46(75.4) | 139(71.3) | 0.530 |
Right coronary artery injury, n (%) | 142(55.5) | 42(68.9) | 107(54.9) | 0.053 |
Circumflex artery injury, n (%) | 106(41.4) | 33(54.1) | 73(37.4) | 0.021 |
Left coronary artery injury, n (%) | 24(9.4) | 8(13.1) | 16(8.2) | 0.92 |
Significant differences between both cohorts in terms of localization of STEMI were not found, even though one vessel injury frequently occurred in MACEs-free patients. In contrast, patients having MACEs represented frequent circumflex artery injury (p = 0.021).
Hemodynamic characteristics in post-STEMI patients at baseline are reported in Table 3. The patients having MACEs had lower LVEF (P = 0.005), higher left atrium volume (P = 0.021), and E/e` ratio (Р = 0.042) than those who had no MACEs.
Variables | Entire STEMI population (n = 256) | Patients who met MACEs (n = 61) | Patients who did not meet MACEs (n = 195) | P value |
HR, per minute | 76±15 | 79±16 | 76±15 | 0.079 |
Systolic BP, mm Hg | 134±25 | 123±22 | 138±25 | 0.003 |
Diastolic BP, mm Hg | 80±12 | 77±12 | 81±12 | 0.019 |
LV EDV, ml | 136±37 | 137±45 | 136±35 | 0.930 |
LV ESV, ml | 64±28 | 69±36 | 63±25 | 0.516 |
LVEF, % | 51±10 | 48±11 | 52±10 | 0.005 |
LA, cm | 4.10±0.51 | 3.68±0.54 | 4.04±0.53 | 0.477 |
LAV, ml | 56±7 | 57±10 | 54±9 | 0.021 |
E/e`, unit | 11.6±4.28 | 12.89±5.34 | 11.31±4.86 | 0.042 |
Spearman correlation analysis between STEMI severity, circulating levels of cardiac biomarkers, co-morbidities and BDNF gene SNP
There were statistically significant correlations of circulating levels of NT-pro-BNP with TIMI score (r = −0.33; p = 0.001), LVEF at baseline (r = −0.42; p = 0.003), obesity (r = −0.26; p = 0.012), age (r = 0.27; p = 0.022), and 66ValVal genotype of BDNF gene (r = −0.41; p = 0.003). The SYNTAX score was inversely correlated with LVEF at baseline (r = −0.34; p = 0.001) and 66ValVal genotype of BDNF gene (r = −0.26; p = 0.001), and positively correlated with T2DM (r = 0.32; p = 0.002).
Univariate and multivariate linear regressions
Univariate linear regression revealed that predictors for the combined clinical endpoints were peak TnI levels, NT-proBNP, SYNTAX score, TIMI score, obesity, LVEF, and genotype 66ValMet+66MetMet in BDNF gene (Table 4). Unadjusted multivariate linear regression showed that the peak of TnI levels, NT-proBNP, SYNTAX score, TIMI score, and 66ValMet+66MetMet genotype in BDNF gene all remained independent predictors for the combined clinical endpoints. After adjustment for SYNTAX score and TIMI score, genotype 66ValMet+66MetMet in BDNF gene (OR = 1.5476; 95% CI = 1.1277 – 4.1426; P = 0.0246) and NT-proBNP (OR = 1.7546; 95% CI = 1.0219 – 3.1002; P = 0.046) independently predicted cumulative clinical endpoint.
Depending variable: combined end point | ||||||||
Variables | Univariate linear regressive analysis (χ2 = 22.917 P = 0.0182) | Multivariate linear regressive analysis(χ2 = 15.244 P = 0.0042) | ||||||
β-coefficient | OR | 95% CІ | Р value | β-coefficient | OR | 95% CІ | Р value | |
Age | 0.03101 | 1.0315 | 1.0000 – 1.0640 | 0.0503 | - | |||
Peak TnI | 0.74033 | 2.0966 | 1.0945 – 4.4990 | 0.0470 | 0.86154 | 1.6132 | 1.0751 – 4.2210 | 0.0480 |
NT-proBNP | 0.8244 | 2.4746 | 1.1233 – 4.6833 | 0.0410 | 0.92370 | 1.8144 | 1.0330 – 3.1122 | 0.0420 |
SYNTAX score | 1.17560 | 1.9428 | 1.2493 – 3.5422 | 0.0244 | 1.41380 | 1.6844 | 1.1830 – 2.3655 | 0.0234 |
TIMI score | 1.37250 | 1.8970 | 0.9720 – 2.880 | 0.0410 | 1.17280 | 1.0940 | 1.010 – 1.3240 | 0.0420 |
BMI > 30 kg/m2 | 1.12320 | 2.1448 | 0.4607 – 3.8995 | 0.0383 | 1.02 | 1.9560 | 0.0774 – 3.4539 | 0.0526 |
Type 2 diabetes mellitus | -0.3267 | 0.7213 | 0.5020 – 1.0364 | 0.0773 | - | |||
Genotype 66ValMet+66MetMet in BDNF gene | 0.74033 | 2.0966 | 1.0945 – 4.4990 | 0.0470 | 0.68301 | 1.9798 | 1.1545 – 4.1065 | 0.0395 |
Smoking | 0.088830 | 1.0929 | 0.5289 – 2.2583 | 0.8104 | - | |||
Anterior STEMI | 0.37969 | 1.4618 | 0.8738 – 2.4455 | 0.1481 | - | |||
LVEF | -0.035797 | 0.9648 | 0.9346 – 0.9961 | 0.0276 | -0.036369 | 0.9643 | 0.9319 – 0.9978 | 0.0574 |
Constant | -2.789400 | - | - | - | - |
Comparison of the predictive abilities of the models
We compared both models (genotype 66ValMet+66MetMet in BDNF gene and NT-proBNP) for STEMI individuals (Table 5). The genotype 66ValMet+66MetMet in BDNF gene was better than NT-proBNP and standard model, while the only adjusted models were entered in the analysis.
Models | Depending variable: combined end point | ||||||||
AUC | NRI | IDI | |||||||
M | 95% CI | Р value | M | 95% CI | P value | M | 95% CI | P value | |
Standard model (TIMI score) | 0.547 | 0.450 – 0.620 | - | Reference | - | - | Reference | - | - |
Genotype 66ValMet+66MetMet in BDNF gene + Standard model vs Standard model | 0.709 | 0.610 – 0.810 | 0.0232 | 0.31 | 0.27 – 0.36 | 0.044 | 0.068 | 0.057 – 0.077 | 0.049 |
NT-proBNP + Standard model vs Standard model | 0.697 | 0.592 – 0.795 | 0.0480 | 0.30 | 0.22 – 0.41 | 0.057 | 0.046 | 0.020 – 0.061 | 0.180 |
Determination of genetic model variants
We found that the model based on the genotype 66ValMet+66MetMet in BDNF gene carried out an additive value because it was defined by the following equation: OR2 = 2 − 1 / OR1 (Table 6). This means that the regression analysis was the best fitted to the model by applying the variables which predicted cumulative clinical endpoint.
ValVal | ValMet | MetMet | |
Patients who met MACEs (n = 61) | 42 | 14 | 5 |
Patients who did not meet MACEs (n = 195) | 148 | 44 | 3 |
OR1 | 1.12 | - | - |
OR2 | 5.23 | - | - |
Kaplan-Meyer analysis for combined endpoint accumulation trends in post-STEMI patients
Kaplan-Meier curves demonstrated that post-STEMI patients having 66ValVal genotype of BDNF gene had the lowest accumulation of combined endpoint when compared with those who had the combination of 66ValMet and 66MetMet genotypes (Cox-criterion, Р = 0.019; log-rank criterion, Р = 0.03) (Figure 2).
Discussion
The results of our study showed negative associations between the 66ValMet + 66MetMet polymorphisms in the BDNF gene and 6-month endpoints in post-STEMI patients. The prognostic significance of BDNF 66ValMet polymorphism was investigated in post-STEMI patients first, while these relations were previously determined in individuals with acute coronary syndrome35. Therefore, there was an association between the BDNF levels and severity of HF NYHA functional class among individuals with HF with reduced LVEF (HFrEF)21, atherosclerosis severity in stable CAD patients36, and patients with unstable angina37. The exact causes for the decline in circulating BDNF levels among patients with known CV diseases (including unstable angina, acute coronary syndrome, STEMI and HF) are uncertain. Probably, BDNF can be released from skeletal muscle, and skeletal muscle dysfunction (rather than loss of skeletal muscle mass) in HF patients may explain lowered levels of circulating BDNF in HFrEF. However, it does not determine declining levels of the peptide in post-STEMI patients with adverse cardiac remodeling, HF with preserved EF, nor recurrent CV events38, 39, 40, 41. Whether BDNF production is under control by myocardial function and exercise is not well-understood. In this context, lowered levels of BDNF have been ensured by SNP in the 66ValMet BDNF gene, which controls synthesis of the peptide42.
Previous animals and clinical trials have revealed that the BDNF levels were modulated by BDNF gene polymorphism and that CV actions of circulating BDNF likely correspond to this SNP43, 44. Moreover, low circulating levels of BDNF were associated with adverse cardiac remodeling after STEMI and higher levels of NTproBNP45. Nevertheless, there are investigations that have yielded no close association between the genetic variants of BDNF and the serum levels of this peptide43, 45. There is a hypothesis that the BDNF Val66Met genotype can affect cardiac function and CV risk through other mechanisms. We found an association between circulating levels of NT-proBNP and BDNF gene polymorphism; however, there is no plausible evidence for the interrelation between BDNF gene polymorphism and cardiac function, severity of atherosclerosis, and CV risk factors (not including T2DM). Importantly, previous clinical studies have shown associations between metabolic disease (such as morbid obesity and T2DM) and circulating levels of the BDNF, but not with the BDNF gene polymorphism46, 47. Some researchers have proposed that adipocytokines can be triggers for the decline of BDNF levels, but BDNF Val66Met polymorphism has shown no significant association with circulating levels of leptin47. However, in our investigations, patients who were enrolled in these studies were not qualified as those having stable CAD or STEMI.
We suggest that BDNF gene polymorphism is an independent factor contributing to MACEs and re-admission to the hospital through altered cardioprotective effect. Since BDNF specifically binds to the tropomyosin-related kinase receptor B, it acts as modulator of various intracellular signaling pathways (e.g. Akt pathway, transforming growth factor-beta/ Smad pathway), which suppress inflammation and oxidative stress, upregulate the viability of ischemic tissue, promote differentiation of endothelial progenitor cells, and initiate neovascularization and angiogenesis in response to hypoxic and ischemia stimuli48, 49, 50, 51. Interestingly, the predictive values for both the BDNF Val66Met gene polymorphism and serum levels of NT-proBNP were almost similar after adjustment for severity of atherosclerosis and STEMI. Although the results of the study demonstrated that the BDNF Val66Met gene polymorphism predicted the combined endpoint in post-STEMI patients, there is no explanation for the exact molecular mechanisms which support this effect. We believe this fact is required to be investigated in the future to clearly understand primary ways that BDNF Val66Met gene polymorphism affects the clinical outcomes of post-STEMI patients after successful PPCI.
Our study had several limitations. The first limitation was associated with the small sample size, it is important to calculate sample size such that the statistical difference between both cohorts can be discerned. However, a large clinical study is required to obtain more information regarding the BDNF gene polymorphism as a predictor for poor clinical outcomes in post-STEMI patients. The second limitation is the lack of optical coherent tomography to rule in correct expanding of stents. The third limitation relates to respectively lowered number of secondary endpoints including hospitalization due to HF, recurrent unstable angina, and STEMI and PPCI. We did not perform analysis of secondary endpoint accumulation.
Conclusion
We found that 66ValMet + 66MetMet BDNF gene polymorphisms independently predicts the combined 6-month endpoint in post-STEMI patients after treatment with PPCI.
Abbreviations
ACEI: angiotensin-converting enzyme inhibitor
ARBs: angiotensin-II receptor antagonist
BDNF: brain-derived neurotrophic factor
BMI: body mass index
BP: blood pressure
CI: confidence interval
CV: cardiovascular
EF: ejection fraction
GFR: glomerular filtration rate
HCE: hypercholesterolemia
HDL: high-density lipoprotein
HF: heart failure
HR: heat rate
LAD: left atrium diameter
LAV: left atrium volume
LDL: low-density lipoprotein
LV: left ventricular
LVEDV: left ventricuar end diastolic volume
LVEF: left ventricular ejection fraction
LVESV: left ventricular end systolic volume
MACEs: major cardiovascular events
MCRA: mineralocorticoid receptor antagonist
OR: odds ratio
PPCI: primary percutaneous coronary intervention
STEMI: ST-segment elevation myocardial infarction
T2DM: type 2 diabetes mellitus
TG: triglycerides
Tn: cardiac troponin
Acknowledgments
We thank all patients participated in the study and staff of the Government Institution “L.T.Malaya Therapy National Institute NAMSU” and the reperfusion center of the “Zaitsev V.T. Institute for General and Emergency Surgery NAMSU” for its assistance in the investigation. There are no previous presentations of the information reported in the article.
Author’s contributions
Olga V. Petyunina: conception of the study, protocol and study design developing, patient enrollment, data collection, statistical analysis, and writing of the article. Mykola P. Kopytsya: data collection, statistical analysis, and writing of the article. Alexander E. Berezin: data collection, statistical analysis, writing of the article and critical revision for intellectual content. All authors read and approved the final manuscript.
Funding
The study is a fragment of the research project: “To study the biochemical, genetic mechanisms of reperfusion damage of the myocardium and to assess the cardioprotective effect of antiplatelet therapy in acute myocardial infarction”, State Registration No. 0117U003028 / Ukraine. No commercial funds were collected for this study.
Availability of data and materials
Data and materials used and/or analyzed during the current study are available from the corresponding author on reasonable request.
Ethics approval and consent to participate
This study was conducted in accordance with the amended Declaration of Helsinki. The institutional review board approved the study (Protocol №8, 29.08.2016), and all participants provided written informed consent.
Consent for publication
Not applicable.
Competing interests
The authors declare that they have no competing interests.
References
-
Karayiannides S, Norhammar A, Frøbert O, James SK, Lagerqvist B, Lundman P. Prognosis in Patients With Diabetes Mellitus and STEMI Undergoing Primary PCI. J Am Coll Cardiol.
2018;
72
(12)
:
1427-1428
.
View Article PubMed Google Scholar -
Paradies V, Zheng H, Chan MHH, Chan MYY, Foo DC, Lee CW, et al. Impact of time of onset of symptom of ST-segment elevation myocardial infarction on 1-year rehospitalization for heart failure and mortality. Am Heart J.
2020;
224
:
1-9
.
View Article PubMed Google Scholar -
Chiara A, Clagnan E, Valent F. Epidemiology and mortality in an Italian region after the adoption of the universal definition of myocardial infarction. J Cardiovasc Med (Hagerstown).
2020;
21
(1)
:
34-39
.
View Article PubMed Google Scholar -
Berezin AE. Circulating Biomarkers in Heart Failure. Adv Exp Med Biol.
2018;
1067
:
89-108
.
View Article PubMed Google Scholar -
El-Ahmadi A, Abassi MS, Andersson HB, Engstrøm T, Clemmensen P, Helqvist S, et al. Acute kidney injury - A frequent and serious complication after primary percutaneous coronary intervention in patients with ST-segment elevation myocardial infarction. PLoS One.
2019;
14
(12)
:
e0226625
.
View Article PubMed Google Scholar -
Halilčević M, Begić E, Džubur A, Šabanović-Bajramović N, Mekić M, Ejubović M, Džubur A, Štimjanin E. Cardiac biomarkers and left ventricular systolic function in acute myocardial infarction with ST- segment elevation in diabetes mellitus type 2 patients. Med Glas (Zenica).
2020;
17
(2)
.
View Article Google Scholar -
Zhang C, Liu H, Wang H, Tao Q, Lin X, Ge S, Zhai Z. The Predictive Value of Potential Hematological Biomarkers in Acute Coronary Syndrome. Clin Lab.
2019;
65
(10)
.
View Article Google Scholar -
Lino DOC, Freitas IA, Meneses GC, Martins AMC, Daher EF, Rocha JHC, Silva Junior GB. Interleukin-6 and adhesion molecules VCAM-1 and ICAM-1 as biomarkers of post-acute myocardial infarction heart failure. Braz J Med Biol Res.
2019;
52
(12)
:
e8658
.
View Article PubMed Google Scholar -
Mzoughi K, Chouaieb S, Zairi I, Fredj S, Ben Kilani M, Berriri S, Zili M, Kraiem S. Prognostic value of ST2 in myocardial infarction. Tunis Med.
2019;
97
(2)
:
335-343
.
-
McGilligan V, Watterson S, Rjoob K, Chemaly M, Bond R, Iftikhar A, et al. An exploratory analysis investigating blood protein biomarkers to augment ECG diagnosis of ACS. J Electrocardiol.
2019;
57S
:
S92-S97
.
View Article PubMed Google Scholar -
Yano H, Chao MV. Neurotrophin receptor structure and interactions. Pharm Acta Helv.
2000;
74
:
253-260
.
View Article Google Scholar -
Skaper SD. The biology of neurotrophins, signalling pathways, and functional peptide mimetics of neurotrophins and their receptors. CNS Neurol Disord Drug Targets.
2008;
7
(1)
:
46-62
.
View Article PubMed Google Scholar -
Kermani P, Hempstead B. Brain-derived neurotrophic factor: a newly described mediator of angiogenesis. Trends Cardiovasc Med.
2007;
17
(4)
:
140-143
.
View Article PubMed Google Scholar -
Nakano I, Kinugawa S, Hori H, Fukushima A, Yokota T, Takada S, et al. Serum Brain-Derived Neurotrophic Factor Levels Are Associated with Skeletal Muscle Function but Not with Muscle Mass in Patients with Heart Failure. Int Heart J.
2020;
61
(1)
:
96-102
.
View Article PubMed Google Scholar -
Emanueli C, Meloni M, Hasan W, Habecker BA. The biology of neurotrophins: cardiovascular function.. Handb Exp Pharmacol.
2014;
220
:
309-328
.
View Article PubMed Google Scholar -
Donovan MJ, Lin MI, Wiegn P, Ringstedt T, Kraemer R, Hahn R, et al. Brain derived neurotrophic factor is an endothelial cell survival factor required for intramyocardial vessel stabilization. Development.
2000;
127
(21)
:
4531-4540
.
-
László A, Lénárt L, Illésy L, Fekete A, Nemcsik J. The role of neurotrophins in psychopathology and cardiovascular diseases: psychosomatic connections. J Neural Transm (Vienna).
2019;
126
(3)
:
265-278
.
View Article PubMed Google Scholar -
Manni L, Nikolova V, Vyagova D, Chaldakov GN, Aloe L. Reduced plasma levels of NGF and BDNF in patients with acute coronary syndromes. Int J Cardiol.
2005;
102
(1)
:
169-171
.
View Article PubMed Google Scholar -
Jiang H, Liu Y, Zhang Y, Chen ZY. Association of plasma brain-derived neurotrophic factor and cardiovascular risk factors and prognosis in angina pectoris. Biochem Biophys Res Commun.
2011;
415
(1)
:
99-103
.
PubMed Google Scholar -
Costa HS, Lima MMO, Figueiredo PHS, Martinelli PM, Camargos ER, Chaves AT, et al. Prognostic value of serum brain-derived neurotrophic factor levels in patients with Chagas cardiomyopathy. Mem Inst Oswaldo Cruz.
2018;
113
:
e180224
.
View Article Google Scholar -
Barman HA, Şahin I, Atıcı A, Durmaz E, Yurtseven E, Ikitimur B, et al. Prognostic significance of brain-derived neurotrophic factor levels in patients with heart failure and reduced left ventricular ejection fraction. Anatolian journal of cardiology.
2019;
22
(6)
:
309-316
.
View Article Google Scholar -
Liu YQ, Su GB, Duan CH, Wang JH, Liu HM, Feng N, et al. Brain derived neurotrophic factor gene polymorphisms are associated with coronary artery disease related depression and antidepressant response. Mol Med Rep.
2014;
10
(6)
:
3247-3253
.
View Article PubMed Google Scholar -
Egan MF, Kojima M, Callicott JH, Goldberg TE, Kolachana BS, Bertolino A,et al. The BDNF val66met polymorphism affects activity-dependent secretion of BDNF and human memory and hippocampal function. Cell.
2003;
112
(2)
:
257-269
.
View Article Google Scholar -
Ibanez B, James S, Agewall S, Antunes MJ, Bucciarelli-Ducci C, Bueno H. et al. ESC Scientific Document Group. 2017 ESC Guidelines for the management of acute myocardial infarction in patients presenting with ST-segment elevation: The Task Force for the management of acute myocardial infarction in patients presenting with ST-segment elevation of the European Society of Cardiology (ESC). Eur Heart J..
2018;
39
(2)
:
119-177
.
View Article PubMed Google Scholar -
Kirby A, Gebski V, Keech AC. Determining the sample size in a clinical trial. Med J Aust.
2002;
177
(5)
:
256-257
.
View Article PubMed Google Scholar -
Sacks DB, Arnold M, Bakris GL, Bruns DE, Horvath AR, Kirkman ME, et al. Guidelines and Recommendations for Laboratory Analysis in the Diagnosis and Management of Diabetes Mellitus. Diabetes Care.
2011;
34
(6)
:
e61-e99
.
View Article PubMed Google Scholar -
Catapano AL, Graham I, De Backer G, Wiklund O, Chapman MJ, Drexel H. et al. ESC Scientific Document Group. 2016 ESC/EAS Guidelines for the Management of Dyslipidemias: The Task Force for the Management of Dyslipidemias of the European Society of Cardiology (ESC) and European Atherosclerosis Society (EAS) Developed with the special contribution of the European Association for Cardiovascular Prevention & Rehabilitation (EACPR). Atherosclerosis.
2016;
253
:
281-344
.
View Article PubMed Google Scholar -
Williams B, Mancia G, Spiering W, Agabiti Rosei E, Azizi M, Burnier M. et al. ESC Scientific Document Group. 2018 ESC/ESH Guidelines for the management of arterial hypertension. Eur Heart J.
2018;
39
(33)
:
3021-3104
.
View Article PubMed Google Scholar -
Ponikowski P, Voors AA, Anker SD, Bueno H, Cleland JGF, Coats AJS. et al. ESC Scientific Document Group. 2016 ESC guidelines for the diagnosis and treatment of acute and chronic heart failure: the task force for the diagnosis and treatment of acute and chronic heart failure of the European Society of Cardiology (ESC). Developed with the special contribution of the Heart Failure Association (HFA) of the ESC. Eur J Heart Fail.
2016;
18
:
891-975
.
View Article PubMed Google Scholar -
Morrow DA, Antman EM, Charlesworth A, Cairns R, Murphy SA, de Lemos JA, et al. TIMI Risk Score for ST-Elevation Myocardial Infarction: A Convenient, Bedside, Clinical Score for Risk Assessment at Presentation. An Intravenous nPA for Treatment of Infarcting Myocardium Early II Trial Substudy. Circulation.
2000;
102
:
2031-2037
.
View Article PubMed Google Scholar -
Eagle KA, Lim MJ, Dabbous OH, Pieper KS, Goldberg RJ, Van WF, Goodman SG, Granger CB, Steg PG, Gore JM, Budaj A, Avezum A, Flather MD, Fox KA. A validated prediction model for all forms of acute coronary syndrome: estimating the risk of 6‐month post-discharge death in an international registry. JAMA.
2004;
291
:
2727-2733
.
View Article PubMed Google Scholar -
Hayase M, Yoda S, Hatta T, Hori Y, Monno K, Fujito H, et al. Prognostic Significance of the Residual SYNTAX Score and Ischemic Reduction Detected with Nuclear Cardiology for Prediction of Major Cardiac Events after Revascularization. Intern Med.
2020
.
View Article PubMed Google Scholar -
Levey AS, Stevens LA, Schmid CH, Zhang YL, Castro AF, Feldman HI, et al, for the Chronic Kidney Disease Epidemiology Collaboration (CKD-EPI) for the CKD-EPI (Chronic Kidney Disease Epidemiology Collaboration). A New Equation to Estimate Glomerular Filtration Rate. Ann Intern Med.
2009;
150
:
604-612
.
View Article PubMed Google Scholar -
Horita N, Kaneko T. Genetic model selection for a case-control study and a meta-analysis. Meta Gene.
2015;
5
:
1-8
.
View Article PubMed Google Scholar -
Kim JM, Stewart R, Kim SY, Kim JW, Kang HJ, Lee JY, et al. Interaction between BDNF val66met polymorphism and personality on long-term cardiac outcomes in patients with acute coronary syndrome. PLoS One.
2019;
14
(12)
:
e0226802
.
View Article PubMed Google Scholar -
Jiang R, Babyak MA, Brummett BH, Hauser ER, Shah SH, Becker RC, et al. Brain-derived neurotrophic factor rs6265 (Val66Met) polymorphism is associated with disease severity and incidence of cardiovascular events in a patient cohort. Am Heart J..
2017;
190
:
40-45
.
View Article PubMed Google Scholar -
Jiang H, Wang R, Liu Y, Zhang Y, Chen ZY. BDNF Val66Met polymorphism is associated with unstable angina. Clin Chim Acta.
2009;
400
(1-2)
:
3-7
.
View Article PubMed Google Scholar -
Caporali A, Emanueli C. Cardiovascular actions of neurotrophins. Physiol Rev.
2009;
89
(1)
:
279-308
.
View Article PubMed Google Scholar -
Sookaromdee P, Wiwanitkit V. Brain-derived neurotrophic factor as biomarker. Anatol J Cardiol.
2020;
23
(3)
:
192
.
View Article PubMed Google Scholar -
Kadowaki S, Shishido T, Honda Y, Narumi T, Otaki Y, Kinoshita D, et al. Additive clinical value of serum brain-derived neurotrophic factor for prediction of chronic heart failure outcome. Heart Vessels.
2016;
31
:
535-544
.
View Article PubMed Google Scholar -
Nakano I, Kinugawa S, Hori H, Fukushima A, Yokota T, Takada S, et al. Serum Brain-Derived Neurotrophic Factor Levels Are Associated with Skeletal Muscle Function but Not with Muscle Mass in Patients with Heart Failure. Int Heart J.
2020;
61
(1)
:
96-102
.
View Article PubMed Google Scholar -
Sustar A, Perkovic MN, Erjavec GN, Strac DS, Pivac N. Association between reduced brain-derived neurotrophic factor concentration & coronary heart disease. Indian J Med Res.
2019;
150
(1)
:
43-49
.
View Article PubMed Google Scholar -
Suriyaprom K, Tungtrongchitr R, Thawnashom K, Pimainog Y. BDNF val66Met polymorphism and serum concentrations of BDNF with smoking in Thai males. Genet Mol Res.
2013;
12
:
4925-4933
.
View Article PubMed Google Scholar -
Terracciano A, Piras MG, Lobina M, Mulas A, Meirelles O, Sutin AR, et al. Genetics of serum BDNF: Meta-analysis of the val66Met and genome-wide association study. World J Biol Psychiatry.
2013;
14
:
583-589
.
View Article PubMed Google Scholar -
Bahls M, Könemann S, Markus MRP, Wenzel K, Friedrich N, Nauck M, et al. Brain-derived neurotrophic factor is related with adverse cardiac remodeling and high NTproBNP. Sci Rep.
2019;
9
(1)
:
15421
.
View Article PubMed Google Scholar -
Krabbe KS, Nielsen AR, Krogh-Madsen R, Plomgaard P, Rasmussen P, Erikstrup C, et al. Brain-derived neurotrophic factor (BDNF) and type 2 diabetes. Diabetologia.
2007;
50
:
431-438
.
View Article PubMed Google Scholar -
Suriyaprom K, Tungtrongchitr R, Thawnasom K. Measurement of the levels of leptin, BDNF associated with polymorphisms LEP G2548A, LEPR Gln223Arg and BDNF Val66Met in Thai with metabolic syndrome. Diabetol Metab Syndr.
2014;
6
:
6
.
View Article PubMed Google Scholar -
Feng N, Huke S, Zhu G, Tocchetti CG, Shi S, Aiba T, et al. Constitutive BDNF/TrkB signaling is required for normal cardiac contraction and relaxation. Proc Natl Acad Sci USA.
2015;
112
(6)
:
1880-1885
.
View Article PubMed Google Scholar -
Hong JH, Park HM, Byun KH, Lee BH, Kang WC, Jeong GB. BDNF expression of macrophages and angiogenesis after myocardial infarction. Int J Cardiol.
2014;
176
(3)
:
1405-1408
.
View Article PubMed Google Scholar -
Halade GV, Ma Y, Ramirez TA, Zhang J, Dai Q, Hensler JG, Lopez EF, et al. Reduced BDNF attenuates inflammation and angiogenesis to improve survival and cardiac function following myocardial infarction in mice. Am J Physiol Heart Circ Physiol.
;
305
(12)
:
H1830-H1842
.
View Article PubMed Google Scholar -
Okada S, Yokoyama M, Toko H, Tateno K, Moriya J, Shimizu I, et al. Brain-derived neurotrophic factor protects against cardiac dysfunction after myocardial infarction via a central nervous system-mediated pathway. Arterioscler Thromb Vasc Biol.
2012;
32
(8)
:
1902-1909
.
View Article PubMed Google Scholar
Comments
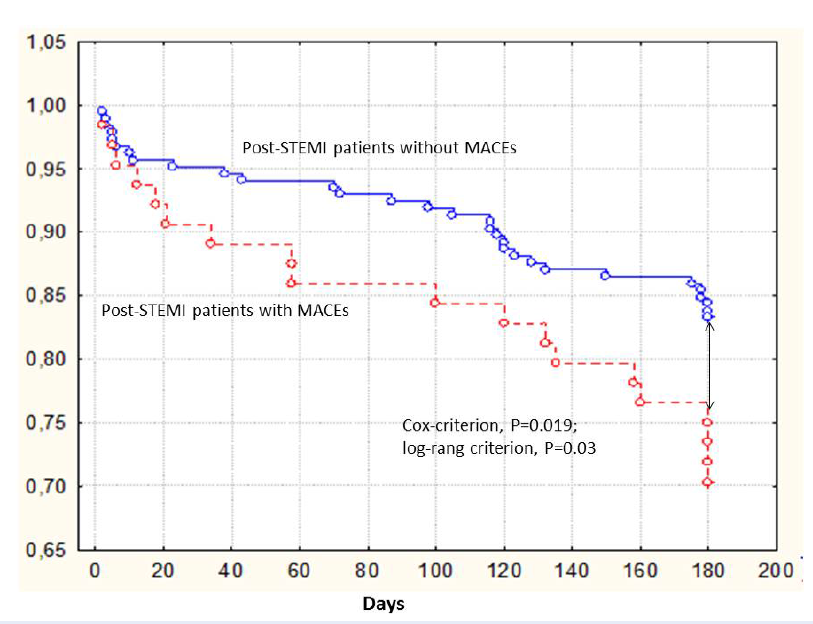
Downloads
Article Details
Volume & Issue : Vol 7 No 8 (2020)
Page No.: 3921-3932
Published on: 2020-08-31
Citations
Copyrights & License

This work is licensed under a Creative Commons Attribution 4.0 International License.
Search Panel
Pubmed
Google Scholar
Pubmed
Google Scholar
Pubmed
Search for this article in:
Google Scholar
Researchgate
- HTML viewed - 6521 times
- Download PDF downloaded - 1438 times
- View Article downloaded - 0 times