Abstract
Autophagy is a natural cellular mechanism in which cellular components such as long-lived proteins and damaged organelles are degraded in response to starvation by forming autophagosomes. Viruses activate the autophagy process, which generates innate immune protection in the host against infection. While the actual molecular mechanism of this contagious viral infection remains unknown, studies on some other betacoronavirus show that their infection of host cells inhibits the autophagy process, resulting in autophagosome accumulation inside the cells. Non-structural protein 6 (NSP6) is crucial in blocking autophagosomes/autolysosome vesicle formation, which are more numerous and smaller than autophagosomes formed upon starvation. Because of its vital role in autophagy, NSP6 can be used as an effective drug target to combat severe acute respiratory syndrome coronavirus 2 (SARS-CoV-2) infections. Therefore, this study aims to detect the mutations in the NSP6 of Indian isolates compared to Wuhan-type isolates. The NSP6 protein of Indiantype SARS-CoV-2 isolates contained 654 point mutations. Furthermore, secondary structure, energy change upon mutation, physicochemical properties, and hydropathy index of wild and mutated proteins were compared, clearly showing that mutations altered NSP6 stability. An immunoinformatics approach was also attempted to identify the B-cell and interferon (IFN)-inducing epitopes for using NSP6 as a probable vaccine candidate. Therefore, this study explored an important drug target (NSP6) essential for autophagy and assembly of coronavirus replicase proteins.
Introduction
Severe acute respiratory syndrome coronavirus 2 (SARS-CoV-2) is a highly transmissible and pathogenic virus that caused this current devastating pandemic disease. The SARS-CoV-2 originated in Wuhan, China, and now can be found in > 210 countries across the globe. The World Health Organization classified SARS-CoV-2 as a pandemic of global concern on March 11, 20201, 2, 3. Since the emergence of this contagious disease, numerous novel studies and several research findings have been published on approaches to curb this pandemic. However, we are still behind in developing effective and durable antiviral therapeutics to combat the COVID-19 outbreak.
SARS-CoV-2 belongs to the family Coronaviridae and order Nidovirales. This virus is supposed to be the third zoonotic coronavirus originating from bats after SARS-CoV and the Middle East respiratory syndrome-related coronavirus (MERS-CoV). However, SARS-CoV-2 is the only novel coronavirus with pandemic potential4, 5, 6, 7, 8. This coronavirus is an enveloped single-stranded RNA virus with a genome of 29,891 nucleotides encoding 9680 amino acids9, 10, 11, 12. The genome of SARS-CoV-2 encodes structural or non structural proteins (NSP1 to NSP16)11, 13, 14. The NSPs are involved in RNA replication and processing, such as the RNA-dependent RNA polymerase (RDRP/NSP12) and helicase (NSP13). However, the functions of some NSPs remain unknown. Among the NSPs, NSP6 is a crucial component of membrane protein (34 KDa), comprising seven transmembrane helices and a conserved C terminus domain. NSP6 induces the autophagy process through the omegasome activation pathway15. NSP6 plays an important role in blocking autophagosomes/autolysosome vesicle formation that are more numerous and smaller than the autophagosomes induced upon starvation16. This condition might be favorable for SARS-CoV-2 to limit the autophagosome delivery of viral components to the lysosome for degradation.
Present study aim to investigate the effect of mutations on the structure of the NSP6 protein from Indian-type SARS-CoV-2 isolates and compare them with the Wuhan-type isolates. Significant alterations were found in the biochemical, immunological, and structural properties of SARS-CoV-2 NSP6 protein. Therefore, this study explored an important protein (NSP6) essential for autophagy and assembly of SARS-CoV-2 replicase proteins.
Methods
Collection of protein sequences
The sequence data for the SARS-CoV-2 NSP6 protein (290 amino acids long) was retrieved from The US National Center for Biotechnology Information (NCBI) virus database. These sequences were filtered for those originating from India from the onset of this disease to August 15, 2022. The Wuhan SARS-CoV-2 virus sequence was used as the reference (accession number: YP_009724389) in the mutational analysis17.
Multiple sequence alignment and mutant identification
NSP6 protein sequences were aligned using CLUSTAL Omega, an online server performing alignment using hidden Markov model profiling18, with the Wuhan-type virus sequence as the reference. Jalview, an alignment viewing software, was used to detect the differences in the NSP6 region with the accession number of the Indian SARS-CoV-2 isolates. The nonsynonymous amino acid substitutions were analyzed using the Protein Variation Effect Analyzer (PROVEAN; v1.1.3) software with a cutoff score of −2.5019. The effect of NSP6 protein mutation on its stability could be inferred from the generated score.
Prediction of NSP6 protein structure
The model of NSP6 protein was predicted using Swiss-Model online web servers. The I-Tasser software was also used to predict the NSP6 protein model. The secondary structure of the NSP6 protein was predicted using the PSIPRED online web tool. The NSP6 protein sequences were examined using the Chou and Fasman secondary structure prediction (CFSSP) online software to detect differences in the secondary structure due to a mutation20. Secondary structure prediction tools were used to analyze alterations in the formation or loss of secondary structures.
Determination of the NSP6 protein’s physicochemical parameters
The NSP6 protein’s physicochemical parameters were determined using ExPASy’s online ProtParam tool. The NSP6 protein’s hydrophobicity was calculated using ExPASy’s Protscale tool21.
Variation in protein stability after mutation
The changes in protein stability after mutation were predicted using the I-Mutant 2.0 online program, which predicts stability in terms of the Gibbs free energy change (ΔG) values, where the negative ΔG values suggest a decrease in flexibility and positive values indicate a gain in flexibility after mutation of the wild-type protein.
B-cell epitope and interferon (IFN)-inducing epitope prediction
The B-cell epitopes of the NSP6 protein were estimated using the Immune Epitope Database (IEDB)22 and Bepipred 2.0 server23. The IFN-inducing epitopes were predicted by IFN epitope24.
T-cell epitope prediction and major histocompatibility complex (MHC) restriction
The IEDB17 Tepitool server was used to predict the T-cell binding epitope and the MHC allele. The binding of processed antigen to an MHC molecule is essential for T-cell recognition and eliciting an immune response.
Determination of the NSP6 protein’s antigenicity and allergenicity
The NSP6 protein’s antigenicity was predicted using the Vaxijen v2.0 server25, and its allergenic properties were determined using the AllerTOP server26.
Results
Collection of target protein sequences for mutational analysis
The NSP6 protein sequences of Indian-type SARS-CoV-2 isolates from the onset of this disease to August 15, 2022, were retrieved from the NCBI database along with the first SARS-CoV-2 virus sequence. In the above period, 548 NSP6 protein sequences were submitted of Indian-type isolates. These sequences were aligned to detect variations in the NSP6 protein region of Indian-type isolates relative to a Wuhan-type isolate. Those mutations in the NSP6 region were recorded with the accession number of the isolate and the mutated base in the wild-type and mutated sequences. The NSP6 region contained 654 point mutations in Indian-type SARS-CoV-2 (Supplementary Table 1). Nine mutations were frequently observed in the NSP6 protein: T77A, V149A, L37F, T181I, V190F, L125F, M183I, and V153C (Supplementary Figure S1).
Nonsynonymous mutations in the SARS-CoV-2 NSP6 protein
Of these 654 mutations, five (I49T, M86I, L142F, I162T, and V190I) were selected for further characterization. The five nonsynonymous amino acid substitutions showed similar effects on the structure of the NSP6 protein. The five NSP6 protein mutants showed a neutral impact at a cutoff PROVEAN score of −2.5 (Table 1).
Amino acid substitution in the helicase region | PROVEAN score | Variation effect on protein |
---|---|---|
I49T | -1.698 | Neutral |
M86I | -0.427 | Neutral |
L142F | -1.244 | Neutral |
I162T | -1.474 | Neutral |
V190I | -0.486 | Neutral |
S.No. | Accession No. | Position of mutation | Wild sequence | Mutated sequence | ΔG | Protein Stability |
---|---|---|---|---|---|---|
1. | QKY59987 | 49 | A | T | -2.80 | Decreases |
2. | QKV26075 | 86 | M | I | -1.02 | Decreases |
3. | QKV26087 | 142 | L | F | -0.66 | Decreases |
4. | QKJ68675 | 162 | I | T | -4.61 | Decreases |
5. | QKJ68699 | 162 | I | T | -4.61 | Decreases |
6. | QKV25895 | 190 | V | I | -0.94 | Decreases |
Physicochemical properties | NSP6 | Amino acid composition | No. | Percent composition (%) |
---|---|---|---|---|
Molecular weight | 33033.69 | Ala (A) | 20 | 6.9 |
No. of amino acids | 290 | Arg (R) | 9 | 3.1 |
Theoretical pI | 9.11 | Asn (N) | 10 | 3.4 |
Instability index | 22.94 | Asp (D) | 8 | 2.8 |
No. of negatively charged (Asp+ Glu) | 11 | Cys (C) | 10 | 3.4 |
No. of positively charged (Arg+ Lys) | 21 | Gln (Q) | 7 | 2.4 |
aliphatic index | 111.55 | Glu (E) | 3 | 1 |
Grand average of hydropathicity | 0.790 | Gly (G) | 15 | 5.2 |
Estimated half-life (mammalian reticulocytes, in vitro) | 1.9 hours | His (H) | 4 | 1.4 |
Atomic composition | Ile (I) | 16 | 5.5 | |
C | 1546 | Leu (L) | 41 | 14.1 |
H | 2378 | Lys (K) | 12 | 4.1 |
N | 360 | Met (M) | 17 | 5.9 |
O | 385 | Phe (F) | 22 | 7.6 |
S | 27 | Pro (P) | 7 | 2.4 |
Formula | C 1546 H 2378 N 360 O 385 S 27 | Ser (S) | 17 | 5.9 |
Total number of atoms | 4696 | Thr (T) | 21 | 7.2 |
Trp (Y) | 17 | 5.9 | ||
Val (V) | 28 | 9.7 | ||
Phy (O) | 0 | 0.0 | ||
Sec (U) | 0 | 0.0 |
S.No. | Start | End | Peptide | Length | Allergenicity | Allergenicity |
---|---|---|---|---|---|---|
1 | 5 | 9 | RTIKG | 5 | (Probable non- antigen) | Non-allergen |
2 | 29 | 34 | TQWSLF | 6 | 0.8722 (Probable antigen) | Non-allergen |
3 | 38 | 41 | YENA | 4 | (Probable non- antigen) | Allergen |
4 | 83 | 89 | MVYMPAS | 7 | -0.0550 (Probable non- antigen) | Allergen |
5 | 95 | 112 | MTWLDMVDTSLSGFKLKD | 18 | 1.3163 (Probable antigen) | Non-allergen |
6 | 131 | 138 | VYDDGARR | 8 | -1.4642 (Probable non- antigen) | Non-allergen |
7 | 152 | 161 | VYYGNALDQA | 10 | 0.5160 (Probable antigen) | Non-allergen |
8 | 172 | 178 | TSNYSGV | 7 | 0.3020(Probable non- antigen) | Non-allergen |
9 | 202 | 204 | ITG | 3 | (Probable non- antigen) | Allergen |
10 | 236 | 267 | RLTLGVYDYLVSTQEFRYMNSQGLLPPKNSID | 32 | 0.6940 (Probable antigen) | Non-allergen |
11 | 275 | 286 | LLGVGGKPCIKV | 12 | 1.2988 (Probable antigen) | Non-allergen |
MHC Restriction of CTL Epitope | |||
---|---|---|---|
T-cell epitope | MHC allele | Antigenicity | Allergenicity |
FAMGIIAMS | HLA-Cw*0401 | 1.1569 (Probable antigen) | Probable nonallergen |
FAMGIIAMS | H2-Db | ||
FAMGIIAMS | H2-Dd | ||
FAMGIIAMS | H2-Kb | ||
FAMGIIAMS | H2-Kd | ||
FAMGIIAMS | H2-Ld | ||
FAMGIIAMS | HLA-G | ||
FAMGIIAMS | H-2Qa | ||
FAMGIIAMS | Mamu-A*01 | ||
CVMYASAVV | HLA-A*2402 | 0.5448 (Probable antigen) | Probable nonallergen |
CVMYASAVV | HLA-B*51 | ||
CVMYASAVV | HLA-Cw*0401 | ||
CVMYASAVV | H2-Db | ||
CVMYASAVV | H2-Dd | ||
CVMYASAVV | H2-Kb | ||
CVMYASAVV | H2-Kd | ||
CVMYASAVV | H2-Ld | ||
CVMYASAVV | HLA-G | ||
CVMYASAVV | H-2Qa | ||
CVMYASAVV | Mamu-A*0 | ||
ALIISVTSN | HLA-A*0203 | 0.8021(Probable antigen) | Probable nonallergen |
ALIISVTSN | HLA-A3 | ||
ALIISVTSN | HLA-A*0301 | ||
ALIISVTSN | HLA-B8 | ||
ALIISVTSN | HLA-Cw*0401 | ||
ALIISVTSN | H2-Db | ||
ALIISVTSN | H2-Dd | ||
ALIISVTSN | H2-Kb | ||
ALIISVTSN | H2-Kd | ||
ALIISVTSN | H2-Ld | ||
ALIISVTSN | HLA-G | ||
ALIISVTSN | H-2Qa | ||
ALIISVTSN | Mamu-A*01 |
Serial No. | Sequence | Method | Result | Score |
---|---|---|---|---|
1 | RLTLGVYDYLVSTQEFRYMN SQGLLPPKNS | SVM based | POSITIVE | 5.3556924 |
2 | LLGVGGKPCIKV | SVM based | NEGATIVE | -0.034460869 |
3 | ITG | SVM based | POSITIVE | 1.084497 |
4 | TSNYSGV | SVM based | NEGATIVE | -0.20288055 |
5 | VYYGNALDQA | SVM based | NEGATIVE | -0.42078445 |
6 | VYDDGARR | SVM based | NEGATIVE | -0.19121745 |
7 | MTWLDMVDTSLSGFKLKD | SVM based | NEGATIVE | -0.12253481 |
8 | MVYMPAS | SVM based | POSITIVE | 0.33703218 |
9 | YENA | SVM based | POSITIVE | 0.87711292 |
10 | TQWSLF | SVM based | POSITIVE | 0.20954355 |
11 | FAMGIIAMS | SVM based | NEGATIVE | -0.53361947 |
12 | CVMYASAVV | SVM based | POSITIVE | 0.25965934 |
13 | ALIISVTSN | SVM based | NEGATIVE | -0.23932506 |
Protein stability detection
The ΔG values for the wild-type and mutated NSP6 proteins were calculated using the I-Mutant 2.0 online server. All mutants showed generally negative and decreasing ΔG values, suggesting a decrease in flexibility in the mutated compared to the wild-type NSP6 protein (Table 2).
Physicochemical parameters and hydropathy of the NSP6 protein
The prediction of the SARS-CoV-2 NSP6 protein’s physicochemical properties showed that it comprises 290 amino acids with a molecular weight of 33033.69 Da, atom number of 4696, aliphatic index of 111.55, instability index of 22.94, and grand average of hydropathy (GRAVY) score of 0.790 (Table 3). The predicted hydropathy plot showed that its N-terminal end contains more hydrophobic residues than its C-terminal end (Figure 1).
NSP6 protein structure prediction
The structure of the NSP6 protein was modeled using the I-Tasser tool since no significant model could be built with the Swiss-Model method. Therefore, the three-dimensional structures were downloaded from the I-Tasser portal (Figure 2 A). The protein model also showed the molecules in close proximity to the NSP6 protein (Figure 2 B). The Prosol server predicted the solubility of the NSP6 protein sequence as 0.4, indicating good solubility (Figure 3). Therefore, the NSP6 protein can be used for vaccine preparations.
Secondary structure of the NSP6 protein
The secondary structure of the NSP6 protein was predicted using the PsiPred server, which inferred the number and position of helix, strand, and coil structures (Figure 4 A). Secondary structures were also predicted using the CFSSP tool. The M86I point mutation showed significant secondary structure changes compared to the wild-type, whereas the other mutants did not impact the secondary structure of the NSP6 protein (Figure 4 B). Replacing methionine with isoleucine leads to the loss of a sheet structure at positions 86, 87, 88, and 89. As a sulfur-containing amino acid, methionine prefers sheet structure and is present in the hydrophobic core of a protein. Therefore, its substitution results in the loss of sheet structure. This variation in the secondary structure of the NSP6 protein can greatly impact viral replication and multiplication.
Assessment of B-cell epitopes in the NSP6 protein
Eleven linear B-cell epitopes were identified in the NSP6 proteins. Their amino acid composition, region of occurrence, antigenicity, and allergenicity are shown in Table 4. In Figure 5, the epitopic regions are shown in yellow, and the non-epitopic regions are shown in green.
T-cell epitope prediction and identification of IFN-inducing epitopes
We identified three T-cell epitopes that have binding ability for different alleles. The sequences of three epitopes were FAMGIIAMS, CVMYASAVV, and ALIISVTSN (Table 5). Moreover, the IFN-inducing epitope server predicted six epitopes positive for IFN-inducing capability, including both B-cell and T-cell epitopes (Table 5). Therefore, these predicted epitopes can induce IFNs for cytokine production.
Prediction of the NSP6 protein’s vaccine properties
In order to become a vaccine candidate, a protein must be immunogenic and non-allergenic. The antigenicity property depends on the vaccine candidate’s ability to bind to both B-cell and T-cell receptors and induce an immune response. Tables 5 and 6 show the immunogenicity of the NSP6 protein. Allergenicity predictions for all epitopes found that only three B-cell epitopes were allergenic; the others were non-allergenic. Therefore, only the non-allergenic epitopes could be used as vaccine candidates (Table 5, Table 6).
Discussion
The emergence of SARS-CoV-2 in late 2019 was reported to be the causal agent of the novel coronavirus pandemic (COVID-19). This contagious virus poses a danger to humans and animals because of its ability to overcome the species barrier and acquire infective properties witnessed in previous SARS-CoV and MERS-CoV outbreaks. SARS-CoV-2 belongs to the RNA virus family, showing enormous genetic variability because of its potential to exhibit a high mutation frequency. Numerous factors are responsible for the transmissibility of SARS-CoV-2 infections across different geographical areas, including poor health and hygiene systems as well as personal hygiene, and climatic and environmental variations27.
Additionally, these RNA viruses can better adapt to an ever-changing environment due to frequent mutations resulting in natural selection. In this study, we detected mutations in the NSP6 protein of Indian isolates because this protein shows an autophagic response and is involved in replicase assembly.
Viruses recruit autophagic cellular machinery to support their rapid transmission and replication28, 29, which can have an antiviral role30. Viruses co-evolve with their usual hosts, strengthening their ability to control autophagic processes to evade host immunity. However, data on the coronavirus autophagy mechanism remain scarce.
Autophagy is a natural cellular mechanism in which malfunctioning cellular components, such as long-lived proteins and damaged organelles, are degraded in response to starvation by forming autophagosomes. Therefore, autophagy is also considered a quality control mechanism operating in the cell28, 29. The macroautophagy process is initiated by a phagophore (a double-membrane structure). The phagophore works by engulfing the substrate molecules destined for degradation and disintegrating inside an autophagosome28, 31. An autolysosome is formed by combining mature autophagosomes with lysosomes, where engulfed cellular material is degraded28, 32.
A previous study showed that the SARS-CoV-2 NSP6 protein inhibits autophagosome expansion after cellular stress such as starvation. Altogether, it acts directly or indirectly to limit autophagosome inhibition of mammalian target of rapamycin (mTOR) signaling via chemicals or upon starvation33.
In this study, we predicted six mutations in the NSP6 region of Indian-type SARS-CoV-2 isolates using a Wuhan-type isolate as the reference. The transmembrane protein structure, secondary structure, and energy change upon mutation were examined, and the physicochemical properties and hydropathy index of the wild-type NSP6 protein were also estimated. An immunoinformatics approach was attempted to identify the NSP6 protein’s T-cell, B-cell, and IFN-inducing epitopes for use as possible vaccine candidates34, 35. Since the NSP6 protein is non-allergenic and antigenic, it represents a possible vaccine target.
Similarly, our findings suggest that six recurrent point mutations affecting the structural stability of NSP6 lie in the open reading frame 10 adjacent region. These mutations arose within the virus’s genome during circulation, affecting its intracellular survival.
The coronavirus NSP6 is a transmembrane protein with a molecular weight of approximately 34 kDa. It localizes to the endoplasmic reticulum membrane and perinuclear space36, 37, 38. Viral replication occurs in double-membrane vesicles (DMVs) that originate from the endoplasmic reticulum and are involved in the formation of replication organelles that protect virus’s genomic RNA from pattern recognition receptors, which can induce an IFN response39, 40. IFN is the first line of defense in response to viral infections, activating innate immunity and promoting adaptive immunity against future infection by the same pathogen41, 42. Xia et al.43 reported that various SARS-CoV-2 non-structural and accessory proteins antagonize IFN-I production in infected host cells. However, mutations in the coronavirus NSP6 protein suppress IFN-I signaling pathways. The structural information may also improve our understanding of NSP6-mediated DMV formation and designing inhibitors of its function. Identifying specific interacting partners will also be vital to understanding the NSP6 antagonism of IFN-I pathways and other functions. Further studies are essential to examine the influence of mutation on important drug targets, such as NSP6, essential for autophagy and assembly of SARS-CoV-2 replicase proteins.
Conclusions
The emergence of the COVID-19 pandemic has caused immense harm worldwide and represents an unprecedented threat to human health and economic losses globally. The rapid development of antiviral therapeutics, such as vaccines, has significantly changed the course of the pandemic and saved millions of lives. However, future variants remain of great concern44. Therefore, it is essential to continue studying coronaviruses, particularly the crucial roles of NSPs like NSP6 in replication.
We have detected six recurrent mutations in the NSP6 protein of Indian-type SARS-CoV-2 isolates. Coronaviruses evolve continuously and rapidly, helping them to adapt to host environments in different areas or locations worldwide. Therefore, they require further investigation. This study’s findings create new avenues for developing therapeutics against one important drug target (NSP6) essential for autophagy and SARS-CoV-2 replicase assembly to halt the transmissibility of this deadly pandemic. While several functions of the SARS-CoV-2 NSP6 protein have been reported, many questions remain unanswered. Therefore, future research should focus on developing a deep understanding of NSP6 protein to design inhibitors to manage the COVID-19 pandemic.
Abbreviations
Da: DaltonDMV: double membrane vesiclesIFN: InterferonskDa: kilodalton NSP6: Non — structural Protein 6
Acknowledgments
None.
Author’s contributions
Niti Yashvardhini designed the experiments and did the analysis, wrote the manuscript, Deepak Kumar Jha did the analysis, wrote the manuscript, Parimal Kumar Khan revised the manuscript, Amit Kumar performed the analysis, Kumar Pranay revised the manuscript. All authors read and approved the final manuscript.
Funding
None.
Availability of data and materials
Data and materials used and/or analyzed during the current study are available from the corresponding author on reasonable request.
Ethics approval and consent to participate
Not applicable.
Consent for publication
Not applicable.
Competing interests
The authors declare that they have no competing interests.
References
-
Huang
C.,
Wang
Y.,
Li
X.,
Ren
L.,
Zhao
J.,
Hu
Y.,
Clinical features of patients infected with 2019 novel coronavirus in Wuhan, China. Lancet.
2020;
395
(10223)
:
497-506
.
View Article PubMed Google Scholar -
Li
Q.,
Guan
X.,
Wu
P.,
Wang
X.,
Zhou
L.,
Tong
Y.,
Early Transmission Dynamics in Wuhan, China, of Novel Coronavirus-Infected Pneumonia. The New England Journal of Medicine.
2020;
382
(13)
:
1199-207
.
View Article PubMed Google Scholar -
Yashvardhini
N.,
Jha
D.K.,
Kumar
A.,
Gaurav
M.,
Sayrav
K.,
Genome sequence analysis of nsp15 from SARS-CoV-2. Bioinformation.
2022;
18
(4)
:
432-7
.
View Article PubMed Google Scholar -
Zhang
Z.,
Wu
Q.,
Zhang
T.,
Pangolin homology associated with 2019-nCoV. bioRxiv 2020.
2020
.
View Article Google Scholar -
Lau
S.K.,
Woo
P.C.,
Li
K.S.,
Huang
Y.,
Tsoi
H.W.,
Wong
B.H.,
Severe acute respiratory syndrome coronavirus-like virus in Chinese horseshoe bats. Proceedings of the National Academy of Sciences of the United States of America.
2005;
102
(39)
:
14040-5
.
View Article PubMed Google Scholar -
Alagaili
A.N.,
Briese
T.,
Mishra
N.,
Kapoor
V.,
Sameroff
S.C.,
Burbelo
P.D.,
Middle East respiratory syndrome coronavirus infection in dromedary camels in Saudi Arabia. mBio.
2014;
5
(2)
:
e00884-14
.
View Article PubMed Google Scholar -
Guan
Y.,
Zheng
B.J.,
He
Y.Q.,
Liu
X.L.,
Zhuang
Z.X.,
Cheung
C.L.,
Isolation and characterization of viruses related to the SARS coronavirus from animals in southern China. Science.
2003;
302
(5643)
:
276-8
.
View Article PubMed Google Scholar -
Jha
D.K.,
Yashvardhini
N.,
Kumar
A.,
Gaurav
M.,
Sayrav
K.,
Structural variations and Immunoinformatics analysis of Orf6 Protein of SARS-CoV-2. Research Journal of Life Sciences, Bioinformatics, Pharmaceutical and Chemical Sciences.
2022;
8
(4)
:
11-22
.
View Article Google Scholar -
Zhu
N.,
Zhang
D.,
Wang
W.,
Li
X.,
Yang
B.,
Song
J.,
Investigating
China Novel Coronavirus,
Team
Research,
A Novel Coronavirus from Patients with Pneumonia in China, 2019. The New England Journal of Medicine.
2020;
382
(8)
:
727-33
.
View Article PubMed Google Scholar -
Cascella
M.,
Rajnik
M.,
Aleem
A.,
Dulebohn
S.C.,
Napoli
R. Di,
Features, Evaluation, and Treatment of Coronavirus (COVID-19). 2022 Oct 13. In: StatPearls [Internet]. Treasure Island (FL): StatPearls Publishing; 2022 Jan. 2022
.
-
Barnard
D.L.,
Coronaviruses: Molecular and Cellular Biology. Future Virology.
2008;
3
(2)
:
119-23
.
View Article PubMed Google Scholar -
Yashvardhini
N.,
Kumar
A.,
Jha
D.K.,
Immunoinformatics Identification of B- and T-Cell Epitopes in the RNA-Dependent RNA Polymerase of SARS-CoV-2. The Canadian Journal of Infectious Diseases & Medical Microbiology.
2021;
2021
:
6627141
.
View Article PubMed Google Scholar -
Jha
D.K.,
Yashvardhini
N.,
Kumar
A.,
Identification of recurrent mutations in exonuclease (nsp14); a potential drug target in SARS-CoV-2. Indian Journal of Pathology & Microbiology.
2021;
64
(4)
:
771-5
.
View Article PubMed Google Scholar -
Yashvardhini
N.,
Kumar
A.,
Jha
D.K.,
Analysis of SARS-CoV-2 mutations in the main viral protease (NSP5) and its implications on the vaccine designing strategies. Vacunas.
2022;
23
:
1-13
.
View Article PubMed Google Scholar -
Cottam
E.M.,
Maier
H.J.,
Manifava
M.,
Vaux
L.C.,
Chandra-Schoenfelder
P.,
Gerner
W.,
Coronavirus nsp6 proteins generate autophagosomes from the endoplasmic reticulum via an omegasome intermediate. Autophagy.
2011;
7
(11)
:
1335-47
.
View Article PubMed Google Scholar -
Cottam
E.M.,
Whelband
M.C.,
Wileman
T.,
Coronavirus NSP6 restricts autophagosome expansion. Autophagy.
2014;
10
(8)
:
1426-41
.
View Article PubMed Google Scholar -
Wu
F.,
Zhao
S.,
Yu
B.,
Chen
Y.M.,
Wang
W.,
Song
Z.G.,
A new coronavirus associated with human respiratory disease in China. Nature.
2020;
579
(7798)
:
265-9
.
View Article PubMed Google Scholar -
Madeira
F.,
Park
Y.M.,
Lee
J.,
Buso
N.,
Gur
T.,
Madhusoodanan
N.,
The EMBL-EBI search and sequence analysis tools APIs in 2019. Nucleic Acids Research.
2019;
47
:
636-41
.
View Article PubMed Google Scholar -
Choi
Y.,
Chan
A.P.,
PROVEAN web server: a tool to predict the functional effect of amino acid substitutions and indels. Bioinformatics (Oxford, England).
2015;
31
(16)
:
2745-7
.
View Article PubMed Google Scholar -
Kumar
T. Ashok,
CFSSP: Chou and Fasman secondary structure prediction server. Wide Spectrum.
2013;
1
(9)
:
15-9
.
View Article Google Scholar -
Gasteiger
E.,
Hoogland
C.,
Gattiker
A.,
Duvaud
S.,
Wilkins
M.,
Appel
R.,
Protein Identification and Analysis Tools on the ExPASy ServerProt Proto Hand 2005.
View Article Google Scholar -
Kim
Y.,
Ponomarenko
J.,
Zhu
Z.,
Tamang
D.,
Wang
P.,
Greenbaum
J.,
Lundegaard
C.,
Sette
A.,
Lund
O.,
Bourne
P.E.,
Nielsen
M.,
Peters
B.,
Immune epitope database analysis resource. Nucleic Acids Res.
2012;
40
(Web Server issue)
:
W525-30
.
View Article Google Scholar -
Larsen
J.E.,
Lund
O.,
Nielsen
M.,
Improved method for predicting linear B-cell epitopes. Immunome Research.
2006;
2
(1)
:
2
.
View Article PubMed Google Scholar -
Dhanda
S.K.,
Vir
P.,
Raghava
G.P.,
Designing of interferon-gamma inducing MHC class-II binders. Biology Direct.
2013;
8
(1)
:
30
.
View Article PubMed Google Scholar -
Doytchinova
I.A.,
Flower
D.R.,
VaxiJen: a server for prediction of protective antigens, tumour antigens and subunit vaccines. BMC Bioinformatics.
2007;
8
(1)
:
4
.
View Article PubMed Google Scholar -
Dimitrov
I.,
Flower
D.R.,
Doytchinova
I.,
AllerTOP--a server for in silico prediction of allergens. BMC Bioinformatics.
2013;
14
(Suppl 6)
:
S4
.
View Article Google Scholar -
Wang
M.A.,
Jiang
L.,
Gong
L.,
Luo
W.,
Guo
C.,
Temperature Significantly Change COVID-19 Transmission in 429 cities. medRxiv 2020.02.22.20025791.
2020
.
View Article Google Scholar -
Choi
Y.,
Bowman
J.W.,
Jung
J.U.,
Autophagy during viral infection - a double-edged sword. Nature Reviews. Microbiology.
2018;
16
(6)
:
341-54
.
View Article PubMed Google Scholar -
Yeganeh
B.,
Ghavami
S.,
Rahim
M.N.,
Klonisch
T.,
Halayko
A.J.,
Coombs
K.M.,
Autophagy activation is required for influenza A virus-induced apoptosis and replication. Biochimica et Biophysica Acta. Molecular Cell Research.
2018;
1865
(2)
:
364-78
.
View Article PubMed Google Scholar -
Lennemann
N.J.,
Coyne
C.B.,
Catch me if you can: the link between autophagy and viruses. PLoS Pathogens.
2015;
11
(3)
:
e1004685
.
View Article PubMed Google Scholar -
Ghavami
S.,
Mutawe
M.M.,
Schaafsma
D.,
Yeganeh
B.,
Unruh
H.,
Klonisch
T.,
Geranylgeranyl transferase 1 modulates autophagy and apoptosis in human airway smooth muscle. American Journal of Physiology. Lung Cellular and Molecular Physiology.
2012;
302
(4)
:
420-8
.
View Article PubMed Google Scholar -
Mehrbod
P.,
Ande
S.R.,
Alizadeh
J.,
Rahimizadeh
S.,
Shariati
A.,
Malek
H.,
The roles of apoptosis, autophagy and unfolded protein response in arbovirus, influenza virus, and HIV infections. Virulence.
2019;
10
(1)
:
376-413
.
View Article PubMed Google Scholar -
Zhou
A.,
Li
S.,
Khan
F.A.,
Zhang
S.,
Autophagy postpones apoptotic cell death in PRRSV infection through Bad-Beclin1 interaction. Virulence.
2016;
7
(2)
:
98-109
.
View Article PubMed Google Scholar -
Jha
D.K.,
Pranay
K.,
Samiksha
Kumar
A.,
Yashvardhini
N.,
The status of COVID-19 vaccines in India: A review. Vacunas.
2023;
24
(3)
:
1576-9887
.
View Article PubMed Google Scholar -
Yashvardhini
N.,
Jha
D.K.,
Kumar
A.,
Sayrav
K.,
Gaurav
M.,
Genetic variations in the Orf7a protein of SARS-CoV-2 and its possible role in vaccine development. Biomedical Research and Therapy.
2021;
8
(8)
:
4497-504
.
View Article Google Scholar -
Lee
J.G.,
Huang
W.,
Lee
H.,
Leemput
J. van de,
Kane
M.A.,
Han
Z.,
Characterization of SARS-CoV-2 proteins reveals Orf6 pathogenicity, subcellular localization, host interactions and attenuation by Selinexor. Cell & Bioscience.
2021;
11
(1)
:
58
.
View Article PubMed Google Scholar -
Ricciardi
S.,
Guarino
A.M.,
Giaquinto
L.,
Polishchuk
E.V.,
Santoro
M.,
Tullio
G. Di,
The role of NSP6 in the biogenesis of the SARS-CoV-2 replication organelle. Nature.
2022;
606
(7915)
:
761-8
.
View Article PubMed Google Scholar -
Snijder
E.J.,
Limpens
R.W.,
Wilde
A.H. de,
Jong
A.W. de,
Zevenhoven-Dobbe
J.C.,
Maier
H.J.,
A unifying structural and functional model of the coronavirus replication organelle: tracking down RNA synthesis. PLoS Biology.
2020;
18
(6)
:
e3000715
.
View Article PubMed Google Scholar -
Scutigliani
E.M.,
Kikkert
M.,
Interaction of the innate immune system with positive-strand RNA virus replication organelles. Cytokine & Growth Factor Reviews.
2017;
37
:
17-27
.
View Article PubMed Google Scholar -
Yang
H.,
Lyu
Y.,
Hou
F.,
SARS-CoV-2 infection and the antiviral innate immune response. Journal of Molecular Cell Biology.
2020;
12
(12)
:
963-7
.
View Article PubMed Google Scholar -
Hoffmann
H.H.,
Schneider
W.M.,
Rice
C.M.,
Interferons and viruses: an evolutionary arms race of molecular interactions. Trends in Immunology.
2015;
36
(3)
:
124-38
.
View Article PubMed Google Scholar -
Kotenko
S.V.,
Durbin
J.E.,
Contribution of type III interferons to antiviral immunity: location, location, location. The Journal of Biological Chemistry.
2017;
292
(18)
:
7295-303
.
View Article PubMed Google Scholar -
Xia
H.,
Cao
Z.,
Xie
X.,
Zhang
X.,
Chen
J.Y.,
Wang
H.,
Evasion of Type I Interferon by SARS-CoV-2. Cell Reports.
2020;
33
(1)
:
108234
.
View Article PubMed Google Scholar -
Gralinski
L.E.,
Menachery
V.D.,
Return of the Coronavirus: 2019-nCoV. Viruses.
2020;
12
(2)
:
135
.
View Article PubMed Google Scholar
Comments
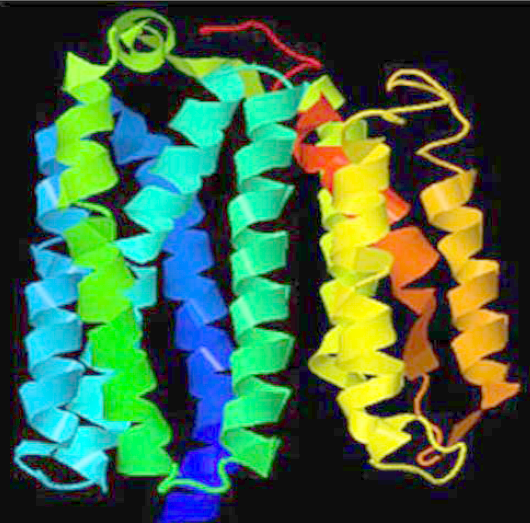
Downloads
Article Details
Volume & Issue : Vol 10 No 7 (2023)
Page No.: 5771-5782
Published on: 2023-07-31
Citations
Copyrights & License

This work is licensed under a Creative Commons Attribution 4.0 International License.
Search Panel
Pubmed
Google Scholar
Pubmed
Google Scholar
Pubmed
Google Scholar
Pubmed
Google Scholar
Pubmed
Search for this article in:
Google Scholar
Researchgate
- HTML viewed - 2969 times
- PDF downloaded - 866 times
- XML downloaded - 98 times
- Supplement downloaded - 627 times